The question ‘When and why do I need artificial intelligence (AI)?’ is being asked more and more frequently as companies look for more efficient ways to optimise their processes. AI can bring decisive advantages, particularly in areas such as demand planning and analysing large amounts of data. In this article, we explain when and why the use of AI makes sense and what benefits it can offer your company.
When and why AI is necessary for weather-dependent products
Weather variations can cause significant fluctuations in demand, specifically for seasonal products, cosmetics, food, and electrical goods. Machine learning algorithms can help companies identify and measure the impact of meteorological factors on sales. Predictive analytics can be used to create "what-if" models for various scenarios.
This approach enables suppliers and retailers to effectively respond to weather-related spikes and dips in demand. Evaluations by our customers show that taking weather effects into consideration (e.g., unusually high temperatures in winter) improves forecast accuracy by 5 to 15 percent for individual food products and by up to 40 percent for other categories of goods.
When and why AI is needed for advertising campaigns
Companies use promotions almost every day to boost their sales. However, analyses of our existing customers have shown that in some cases 25 to 50 percent do not lead to a noticeable increase in sales.
This is also confirmed by a study by Nielsen, a leading market research company, which states that around 60 per cent of advertising campaigns do not cover their costs or lead to additional costs rather than profit.
Paradoxically, the same percentage also appears in a report by Gartner, Here, however, it applies to companies that still plan their promotions on the basis of Excel or spreadsheets and thus try to forecast their impact.
It is to be assumed that enterprises without suitable IT solutions can hardly provide reliable prognoses for costly marketing campaigns. Again, this may be because results of promotions depend on numerous factors and complicated relationships hidden in large amounts of raw data. Fortunately, machine learning can handle this challenging task as well.
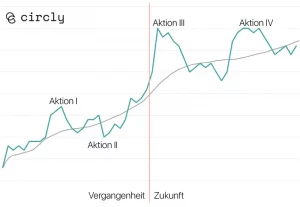
In summary, improvements in promotional forecasting have two immediate benefits. First, they prevent marketing teams from spending too much money on promotions that don't pay off. Second, they lead to more accurate inventory management that eliminates the risk of overstocking or "under stocking".
Wann und warum KI bei variabler Nachfrage von Vorteil ist
A variety of changing variables is the most common reason why forecasts are inaccurate. A highly variable market, numerous factors influencing buying behavior, and a multitude of data points often make demand planning too complex to be successfully accomplished with simple tools such as Excel spreadsheets.
The resulting high complexity of the supply chain, short-term demand spikes, and the resulting cost of misplanning are a suitable reason to move from spreadsheets and manual databases to an ML-powered planning system with advanced predictive capabilities.
It allows waste to be reduced by up to 90 % without compromising service quality and enables rapid responses to potential bottlenecks.
Praxisbeispiel: Der Nutzen von KI für Predictive Sales Analytics
The use of machine learning in combination with neural networks involves some trade-offs in its own implementation. Depending on the planning horizon, data availability and task complexity, different statistical, ML and AI solutions can be used. This also depends on the use case and the objective. A concrete example could be in the area of predictive sales analytics.
Predictive Sales Analytics: Intervening the Future
One of the most common operational applications of machine learning combined with statistical methods is predictive analytics.
It allows you not only to estimate demand, but also to gain an understanding of what will drive sales and how customers are likely to behave under certain conditions. To find out what might happen in the future.
In this context, data preparation is one of the main tasks, because the rule of "garbage in garbage out" which means "if you put bad data in, you won't get anything else out of it" always applies when creating data models. But this is where external help can help out.
For example, services or solutions such as Circly's predictive analytics software perform the following tasks:
• Aggregation of historical and new data from various internal and external sources such as sales figures, marketing campaigns, weather, Google, etc
• Data cleansing;
• Determining which forecasting algorithm best fits your product;
• Building predictive models to identify likely outcomes and discover relationships between different factors;
• Monitoring the models to measure their business results and improve predictive accuracy.
A predictive analytics tool allows you to combine business information with key economic indicators, advertising activity, weather changes, and other factors that influence customer preferences and purchasing decisions. It makes it easier to identify new market opportunities and generates more detailed insights into future demand.
Since predictive analytics is not the simplest technology due to complex machine learning algorithms and neural networks (AI), it is advisable to consult experts or buy in existing and specialized solutions like Circly's.
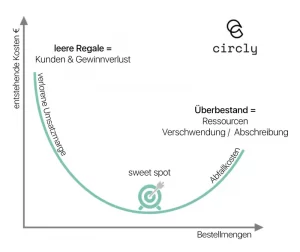
Added value: finding the sweet spot using predictive analytics.
The most difficult part of planning processes such as purchasing or production planning is the realistic estimation of quantities. This is usually done on the basis of empirical values and evaluations. The justified uncertainty that arises from planning too little and thus annoying the customer often leads to a large safety stock.
Products that are not sold then usually end up in the garbage can and are written off. With the help of predictive analytics tools, this can be avoided because a wide variety of factors can be integrated and there is no reason for uncertainty because the real demand can be predicted very precisely.
Human abilities remain important
Demand forecasting is a challenging task that still offers much potential for optimization. Currently, less than 30 percent of business transactions are predicted accurately.
As mentioned, the use of machine learning tools or AI can narrow the gap between prediction and real demand. However, this does not mean that every company should immediately undertake complex development projects to create their own software.
A machine learning module to make data-driven changes to near-term planning can already add value. Circly, for example, offers full-function systems as well as the ability to make small enhancements to your existing system. This allows solving those problems which are difficult to solve with traditional methods in a simple and easy way.
No matter how advanced a prediction solution may be, the most important decisions still are up to humans themselves. Even to create artificial intelligence, it requires an industry specialist to define what factors should be considered in the forecasting model. Human logic is required to evaluate the relevance of the results generated by digital "brains" and to draw final conclusions based on common sense and deep domain knowledge.
For this reason, Circly systems include a collaborative approach that allows various interactions to be included in the forecasting process. Only when the best of both worlds, artificial and human intelligence can be utilized, optimal results can emerge and valuable resources can be saved.