What is the biggest "pain point" for executives in companies?
Gartner,the world's leading IT research firm, gives a clear answer: demand volatility.
To many factors - starting with weather variability and ending with posts from social media influencers - impact buyers and cause them to change their minds frequently. Making the situation even worse, things that change shoppers' intentions happen quite unexpectedly.
Consider, for example, teenage climate activist Greta Thunberg. Her protest to stop flying for environmental reasons sparked the "Flight Shame" movement.
There is no magic wand to predict scenarios like the "Thunberg effect." But there are technologies to enhance the accuracy of demand forecasting. Frankly, it will never be 100 percent accurate, but they are accurate enough to help you as a business achieve your business goals.
In this blog post, we will outline the capabilities of current forecasting methods.
The importance and significance of forecasts in demand and supply planning
Wie Bedarfsprognosen die Effizienz steigern: Grundlagen und Methoden
Demand forecasting is the process of estimating the likely future demand for a product or service. The phrase is often used used interchangeably with demand planning, but the latter is a more broad process that begins with, but is not limited to, forecasting.
According to the Institute of Business Forecasting and Planning (IBF), demand planning "uses forecasts and experience to estimate demand for various items at different points in the supply chain." In addition to estimates, demand planners participate in inventory optimization, ensuring the availability of needed products and monitoring the gap between forecasts and actual sales.
Demand planning is the launch pad for many other activities, such as warehousing, distribution, price forecasting, and especially supply planning, which aims to meet demand and requires data on what customers are likely to want.
Here we return to forecasting. Being as close to reality as possible is key to improving efficiency throughout the supply chain. But how do you achieve the highest possible accuracy? The answer depends on the type of company, the available resources and the objectives.
So let's compare the available options: traditional statistical forecasting, machine learning algorithms, predictive analytics that combines both approaches, and demand sensing as a supporting tool.
Classic statistical forecasts:
suitable for stable markets, but not for changes.
Traditional statistical methods (TSM) have been around for a long time and are still an integral part of forecasting processes in production and retail.
The only difference to earlier calculations is that they are now performed automatically by software solutions. For example, time series forecasts for sales and trends can be created in Excel.
To forecast the future, statistics uses data from the past. This is why statistical forecasts are often referred to as "historical analysis." Traditional forecasts are still the most popular method of predicting sales. Typically, demand planning solutions based on statistical techniques can be executed in Excel and existing enterprise resource planning (ERP) systems seamlessly, without the need for additional technical expertise. The most advanced systems may consider seasonality and market trends, as well as apply numerous methods to fine-tune results.
It should be noted that an important requirement for statistical forecast accuracy is the stability of the market. The analysis assumes that history repeats itself: situations that occurred two or three years ago will repeat themselves.
Demand patterns that can be measured well statistically:
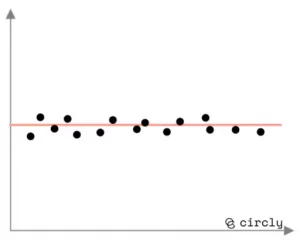
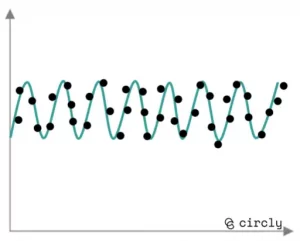
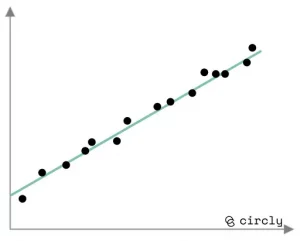
However, this is far from the reality. Statistical methods fail to predict illogical changes in customer preferences or to predict when market saturation will happen.
In summary, automated statistical forecasting provides a sufficient level of accuracy for:
-
- medium to long term planning,
-
- well established items that have a stable demand, and
-
- Forecasting overall demand
-
- Is not suitable for sales planning of individual stock keeping units (SKUs).
So, does it possibly make sense to invest in more sophisticated technologies, such as machine learning or artificial intelligence, after all?
Machine Learning for Demand Planning:
Enhanced Accuracy at the Cost of Added Complexity.
Increased computer power on the one hand and increased demand volatility on the other created the perfect conditions for increased use of machine learning (ML) for demand planning.
Building on the statistical models already presented, machine learning leverages additional internal and external data sources to make more accurate, data-driven predictions. So-called "ML engines" can work with both structured and unstructured data, such as past sales reports (historical data), marketing campaigns, macroeconomic indicators, social media signals (retweets, shares, etc), weather forecasts and more.
But the use of machine learning is worthwhile. It applies automated and complex mathematical algorithms to recognize a wide variety of patterns, detect demand signals, and identify complicated relationships in large data sets without intervention.
Intelligent systems not only analyze massive amounts of data, but continuously retrain models and adapt to changing market conditions to compensate for volatility. These capabilities enable ML-based software to produce more accurate and reliable forecasts in complex scenarios.
As a result, it's no surprise that companies that have added machine learning to their existing systems have reported a 5 to 15 percent increase in forecast accuracy (up to 85 and even 95 percent accuracy). In addition, the efficiency of the deploying company was increased due to the elimination of time-consuming manual adjustments and recalibrations.
To reap the benefits of machine learning, sufficient computing power and large volumes of high-quality data are required. Otherwise, the system will not be able to learn and generate valuable predictions. Furthermore, you should also consider the added complexity in terms of maintaining the software and interpreting the results. While ML models reach conclusions without human intervention, an expert must determine which factors (called features) are fed into the model, which of them have the greatest impact on predictive power, and why the model generates a particular prediction.
All of this, when you implement such a project yourself, can drive up your expenses for infrastructure and personnel. So you should make sure that the optimization of accuracy, cover the associated expenses or hire a technology partner like Circly to handle the aforementioned tasks.
Companies like Circly not only take care of the required infrastructure, creation of models and maintenance, but also constantly optimize the models to achieve the best results.
Here's an overview of when machine learning definitely works better than traditional statistics:
-
- short- to medium-term planning,
-
- volatile demand patterns,
-
- rapidly changing conditions & trends,
-
- new product launches.
Traditional Forecasts | Machine Learning Forecasts | Circly's AI Forcast | |
---|---|---|---|
How many variables and data sources can be factored in? | Adding additional variables and sources requires significant effort. | Multiple variables & sources can be easily integrated thanks to the high level of automation. | A variety of variables and provided data can be easily integrated. |
Extent of manual work: | High | Low | Low |
Amount of data required: | Low | Large | Medium to Large |
Maintenance effort: | Low | High | Low |
Technology requirements: | Low | High | Low to Medium |
Best fit: | Long term planning Established products Stable demand |
Medium term planning New products Volatile demand scenarios |
Medium & short term planning Changing factors Existing processes |
Do you have questions about possible areas of use?
Contact us and arrange your individual and non-binding meeting with our management. und vereinbaren Sie sich Ihr individuelles und unverbindliches Gespräch mit unserer Geschäftsführung.